Remember Reinforcement Learning? It's Never Been More Relevant
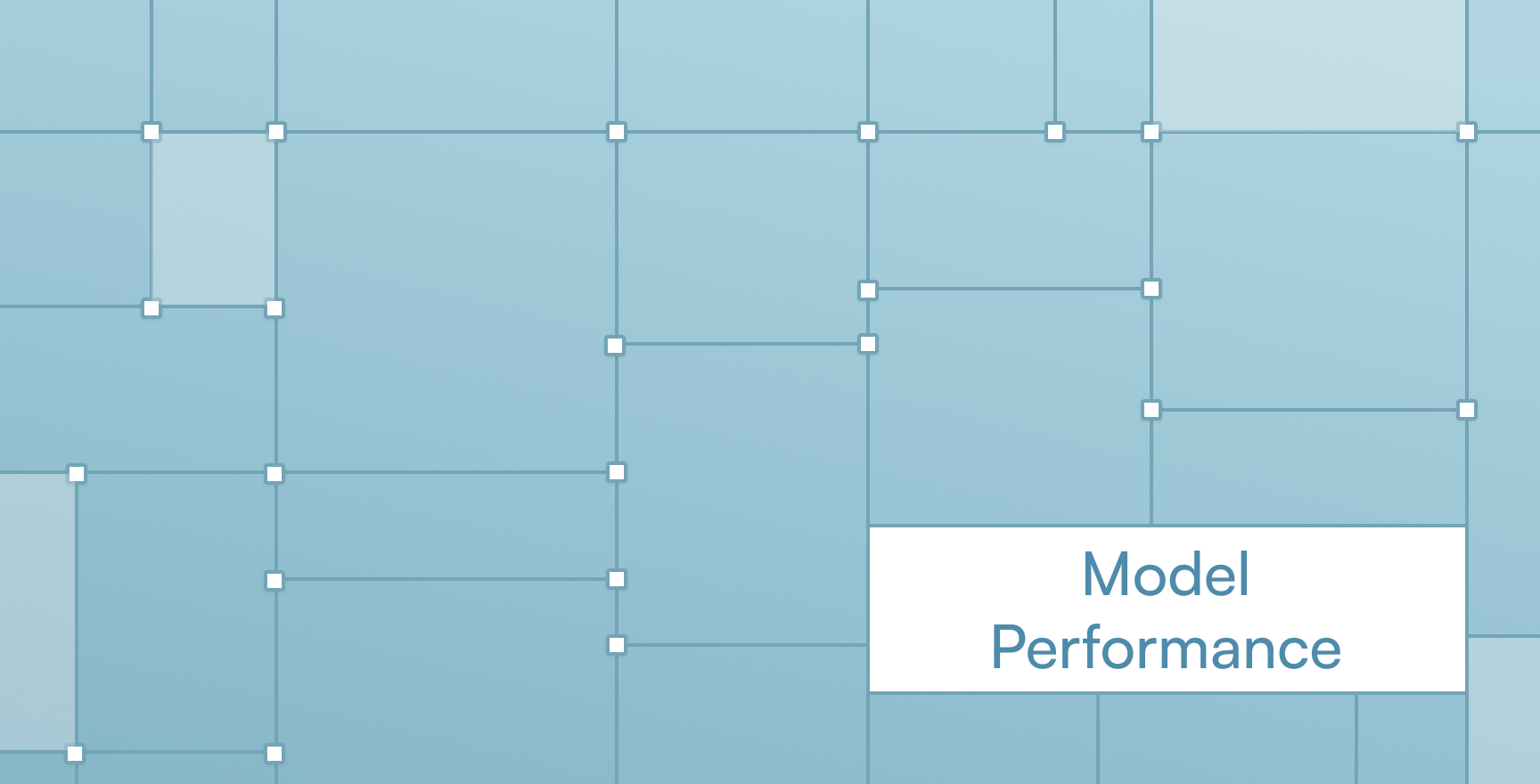
The Reinforcement Learning Renaissance
The machine learning community is abuzz with terms like "RLHF" and "alignment" as reinforcement learning shapes today's most powerful LLMs. While RL's application to language models is revolutionary, it's essential to recognize that reinforcement learning has been a cornerstone of AI development for decades.
At Runloop.ai, we've been implementing reinforcement learning techniques to perfect the performance of coding agents. Let's post examine RL's historical significance, diverse applications, and why it's now central to generative AI development.
RL's Technical Foundations: A Brief History
Reinforcement learning's origins trace back to the 1980s and early 1990s, when researchers like Richard Sutton and Andrew Barto developed its mathematical foundations. Their book "Reinforcement Learning: An Introduction" (first published in 1998, second edition in 2018) remains the field's definitive text.
Key technical milestones include:
- 1989: Chris Watkins formalizes Q-learning, enabling agents to learn optimal policies without environment models
- 1992: Gerald Tesauro's TD-Gammon demonstrates RL's potential by achieving expert-level backgammon play through self-training
- 2013: DeepMind combines deep neural networks with Q-learning to create Deep Q-Networks (DQN), mastering Atari games
- 2015-2016: AlphaGo defeats world champion Lee Sedol, using RL techniques including Monte Carlo Tree Search
- 2018: OpenAI introduces PPO (Proximal Policy Optimization), now the standard algorithm for RLHF in LLMs

Real-World Applications: Where RL Excels
While LLM tuning dominates current discussions, reinforcement learning has been transforming multiple industries:
Robotics and Automation: Companies like Boston Dynamics use RL to train robots for complex physical tasks. Their Atlas robot learned parkour movements through reinforcement learning, something impossible with traditional programming. Similarly, Runloop's RoboFlow platform uses RL algorithms to optimize robotic assembly processes, reducing training time by 54% compared to conventional methods.
Autonomous Systems: Waymo's self-driving vehicles leverage RL for complex decision-making in unpredictable traffic scenarios. Their vehicles have logged over 20 million miles as of January 2024, with reinforcement learning handling edge cases traditional rule-based systems couldn't address.
Resource Management: Google reduced data center cooling costs by 40% using RL systems that dynamically adjust cooling parameters. Microsoft achieved similar results with RL-powered HVAC optimization across their campus in 2023.
Why RL Shines in LLM Development
Reinforcement learning provides the crucial bridge between raw language model capabilities and human-aligned outputs. The technical implementation typically follows this workflow:
- Base Model Training: Train a foundation model using standard next-token prediction (e.g., GPT-4, Claude, Gemini)
- Reward Modeling: Human evaluators rate model outputs, creating a dataset to train a reward model
- RL Fine-tuning: Apply algorithms like PPO to optimize the model against the reward function
A concrete example from Anthropic's Constitutional AI approach (December 2022) demonstrated how RL fine-tuning significantly reduced harmful outputs while maintaining or improving helpfulness metrics.
The Technical Future of RL
As we look forward, reinforcement learning will continue expanding beyond current applications. Emerging areas include:
- Multi-agent reinforcement learning for complex system optimization
- Offline RL for scenarios where online exploration is impractical
- RL-powered digital twins for infrastructure management and planning
The surge in LLM-focused RL applications represents not a new technology, but the maturation of reinforcement learning into mainstream AI development. For technical teams looking to implement RL solutions, understanding this rich historical context provides valuable perspective on this powerful, evolving discipline.
Scale your AI Infrastructure
solution faster.
Stop building infrastructure. Start building your AI engineering product.