LLM Fine-Tuning Methods: A Complete Guide to Post-Training Optimization Techniques
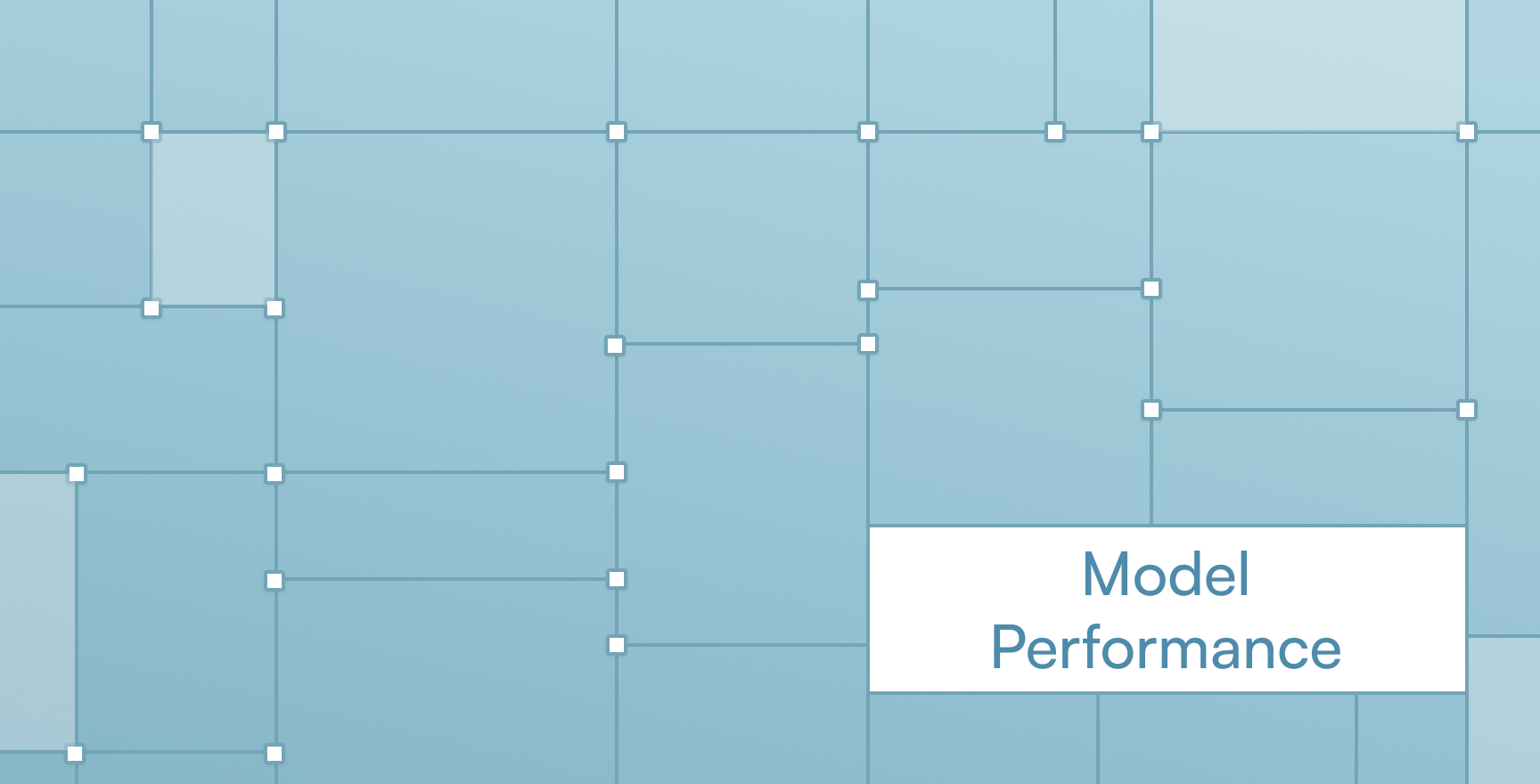
Fine-Tuning Large Language Models: A Comprehensive Guide to Post-Training Methods
AI developers constantly seek ways to refine large language models (LLMs) to improve their performance, efficiency, and alignment with human intent. While pre-training lays the foundation, post-training fine-tuning is where models are truly optimized for real-world applications. Understanding the nuances of fine-tuning methods can help developers create more reliable and scalable AI systems.
In this article, we will briefly discuss pre-training and post-training, then dive deeply into different post-training fine-tuning techniques, including Supervised Fine-Tuning (SFT), Reward Fine-Tuning (RFT), Reinforcement Learning with Human Feedback (RLHF), Contrastive Learning (CoCoMix), LoRA (Low-Rank Adaptation), and Adapter-Based Fine-Tuning. Understanding these methods is essential for anyone looking to improve the performance of LLMs for specific use cases.
Pre-Training vs. Post-Training
Pre-Training: The Foundation
Pre-training is the initial phase of LLM development. It involves training a model on a massive dataset of text (e.g., books, websites, and articles) using self-supervised learning objectives such as:
- Masked Language Modeling (MLM): The model predicts missing words in a sentence.
- Causal Language Modeling (CLM): The model generates the next token in a sequence.
- Next Sentence Prediction (NSP): The model determines if one sentence follows another logically.
Pre-training is computationally expensive and requires high-resource GPUs and TPUs. While it provides a solid linguistic foundation, it lacks domain-specific knowledge and alignment with human intent, necessitating post-training.
Post-Training: Refining and Aligning the Model
Post-training enhances a pre-trained model’s performance by fine-tuning it on specialized data, improving safety, factual accuracy, and task-specific abilities. This phase includes various fine-tuning methods, which we will explore in depth.
Post-Training Fine-Tuning Methods
1. Supervised Fine-Tuning (SFT)
What It Is
Supervised Fine-Tuning (SFT) involves training an LLM on labeled datasets consisting of (input, output) pairs. This method is effective for improving task-specific performance, such as chatbots, summarization, and code generation.
How It Works
- The model is initialized with pre-trained weights.
- It is fine-tuned using a labeled dataset with correct responses.
- The optimization process minimizes the loss between the model's predictions and the ground truth.
Pros and Cons
✅ Simple to implement and improves accuracy on specific tasks.✅ Can be used for domain adaptation (e.g., legal, medical AI).❌ Limited by dataset quality—biases in data can affect outcomes.❌ Does not directly optimize for human preferences.
2. Reward Fine-Tuning (RFT)
What It Is
Reward Fine-Tuning (RFT) trains an LLM to generate responses that are preferred by humans or align with predefined objectives. It often follows SFT to improve alignment with user expectations.
How It Works
- A reward model is trained using human feedback on multiple responses.
- The LLM is fine-tuned using reinforcement learning techniques (e.g., Proximal Policy Optimization, PPO) to maximize the reward score.
Pros and Cons
✅ Leads to more helpful and human-aligned responses.✅ Reduces harmful or biased outputs.❌ Requires a well-designed reward function.❌ Can be computationally expensive.
3. Reinforcement Learning with Human Feedback (RLHF)
What It Is
RLHF is an advanced version of RFT that uses human-in-the-loop training to optimize an LLM’s performance. It is widely used in models like ChatGPT to align responses with user preferences.
How It Works
- Step 1: Collect Human Preferences – Human annotators rank different model responses.
- Step 2: Train a Reward Model – The rankings train a reward model that predicts human preferences.
- Step 3: Fine-Tune Using Reinforcement Learning – The base model undergoes RL optimization to maximize the reward function.
Pros and Cons
✅ Enhances response coherence and reduces harmful outputs.✅ Makes the model more aligned with human values.❌ Requires human labor for data collection.❌ Can introduce bias if the feedback is not diverse.
4. Contrastive Conditional Mixture (CoCoMix)
What It Is
CoCoMix improves instruction following by incorporating contrastive learning and mixture models.
How It Works
- The model learns by comparing good and bad responses.
- It uses contrastive loss to differentiate high-quality vs. low-quality responses.
- The mixture of experts helps the model generalize across diverse prompts.
Pros and Cons
✅ Increases response diversity and quality.✅ Helps distinguish between factual and misleading content.❌ More complex than standard fine-tuning.❌ Requires carefully curated contrastive datasets.
5. LoRA (Low-Rank Adaptation)
What It Is
LoRA is a parameter-efficient fine-tuning method that reduces computational costs by adapting only a subset of model parameters.
How It Works
- Instead of updating all parameters, LoRA adds trainable low-rank matrices that modify only certain layers.
- It maintains the pre-trained weights while introducing small, efficient updates.
Pros and Cons
✅ Drastically reduces fine-tuning costs.✅ Allows adaptation without modifying the entire model.❌ Less effective for extreme domain shifts.❌ Limited ability to correct foundational model flaws.
6. Adapter-Based Fine-Tuning
What It Is
Adapter modules are lightweight neural layers added to an existing model to enable domain adaptation without full retraining.
How It Works
- Instead of modifying the entire LLM, adapter layers are inserted into certain transformer layers.
- These adapters are fine-tuned on specialized datasets, improving task-specific performance.
Pros and Cons
✅ Faster and cheaper than full fine-tuning.✅ Allows multi-domain adaptation without storing multiple models.❌ Requires careful design to balance between generalization and specialization.
Choosing the Right Fine-Tuning Method
Fine-tuning is essential for adapting LLMs to real-world applications. Supervised Fine-Tuning (SFT) serves as the foundation, while Reward Fine-Tuning (RFT) and RLHF help improve response alignment. CoCoMix enhances model robustness, and LoRA/Adapters provide efficient fine-tuning alternatives.
As AI continues to evolve, choosing the right fine-tuning approach will be crucial in ensuring that LLMs are accurate, aligned, and efficient. Understanding these methods allows developers to refine and optimize AI models to better serve users across different industries.
Scale your AI Infrastructure
solution faster.
Stop building infrastructure. Start building your AI engineering product.