RAG in an Era of Fine-Tuning: Understanding RAFT's Evolution
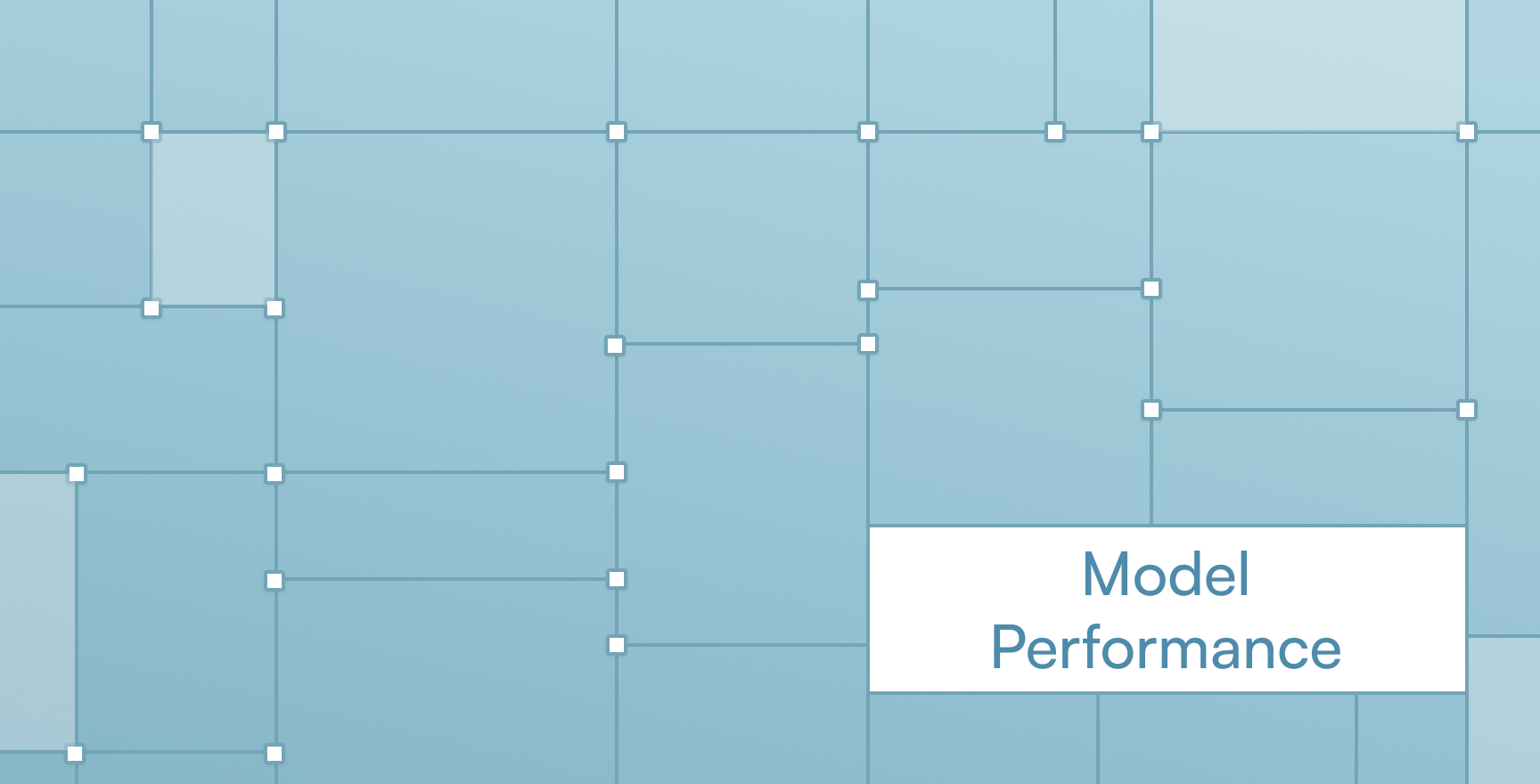
The Evolving Landscape: RAG vs. Fine-Tuning
As large language models (LLMs) continue to advance, the challenge of adapting them to specialized domains becomes increasingly important. Two primary approaches have emerged to address this challenge:
Retrieval-Augmented Generation (RAG) enhances LLMs by integrating external knowledge sources during inference. Operating under a "retrieve-and-read" paradigm, RAG fetches relevant documents based on input queries, which the model then uses to generate contextually-enriched responses. While powerful for accessing up-to-date information, traditional RAG systems face a fundamental limitation: the LLM itself hasn't been trained to effectively identify, prioritize, or use domain-specific knowledge.
Fine-tuning, conversely, adapts pre-trained LLMs through additional training on specialized datasets. This approach excels at teaching models domain-specific patterns and output formats but falls short when information evolves—the knowledge remains static until the next fine-tuning session. As one researcher aptly described it, fine-tuning is "like memorizing documents and answering questions without referencing them during the exam."
Both approaches have clear trade-offs. RAG offers knowledge flexibility but lacks domain-specific reasoning capabilities. Fine-tuning provides specialized knowledge integration but struggles with evolving information and external knowledge retrieval.
Enter RAFT: The Best of Both Worlds
Retrieval-Augmented Fine-Tuning (RAFT) emerges as a groundbreaking solution that combines the strengths of both approaches. RAFT trains models specifically to leverage domain-specific knowledge while maintaining the ability to work with external information sources.
The Mechanics of RAFT
At its core, RAFT introduces a novel approach to preparing fine-tuning data. Each training example consists of:
- A question (Q) - The query that needs answering
- A set of documents (Dk) - Deliberately including both:
- "Oracle" documents containing relevant answers
- "Distractor" documents with irrelevant information
- A chain-of-thought style answer (A)* - Generated from oracle documents with detailed reasoning
This structured approach teaches the model two critical skills simultaneously:
- How to identify and prioritize relevant information
- How to construct well-reasoned answers from domain-specific content
The training dataset also incorporates a balance between:
- Questions with both oracle and distractor documents (percentage P)
- Questions with only distractor documents (percentage 1-P)
This balanced approach prepares the model to handle both scenarios—when relevant information is available and when it must rely on its pre-trained knowledge.
Why RAFT Outperforms Traditional Approaches
Research has demonstrated that RAFT consistently outperforms both standard fine-tuning and traditional RAG implementations across multiple specialized domains, including:
- Medical literature (PubMedQA)
- Software documentation (HuggingFace, TensorFlow Hub)
- Multi-hop reasoning tasks (HotpotQA)
RAFT's success can be attributed to its ability to teach models not just what domain-specific information looks like, but how to effectively retrieve, prioritize, and reason with it—even when faced with distracting or irrelevant content.
Implementing RAFT in Practice
For technical teams looking to implement RAFT, several key considerations emerge from the research:
- Chain-of-Thought Integration: Ablation studies show that including chain-of-thought reasoning in training examples significantly improves performance across most datasets.
- Optimal Document Balance: Finding the right ratio between oracle and distractor documents (typically around 80% oracle inclusion) creates the most robust models.
- Distractor Management: The optimal training configuration typically involves one oracle document with four distractor documents—enough to challenge the model without overwhelming it.
RAFT's Place in the Modern LLM Ecosystem
As domain-specific applications continue to grow in importance, RAFT represents a significant advancement in LLM adaptation techniques. By bridging the gap between traditional fine-tuning and RAG, it addresses the limitations of each while preserving their respective strengths.
For teams working with domain-specific knowledge bases, RAFT offers a path to create more accurate, efficient, and robust AI systems. The technique is particularly valuable for industries where specialized knowledge is critical but constantly evolving—such as healthcare, legal, and technical documentation.
The Future of Domain-Specific LLMs
RAFT demonstrates that the future of domain adaptation isn't about choosing between fine-tuning or retrieval augmentation—it's about intelligently combining these approaches. As LLMs continue to evolve, techniques like RAFT that teach models not just what to know but how to effectively find and use information will become increasingly important.
For organizations building domain-specific AI solutions, understanding and implementing RAFT could provide a substantial competitive advantage—creating models that combine the flexibility of RAG with the specialized capabilities of fine-tuning.
Scale your AI Infrastructure
solution faster.
Stop building infrastructure. Start building your AI engineering product.