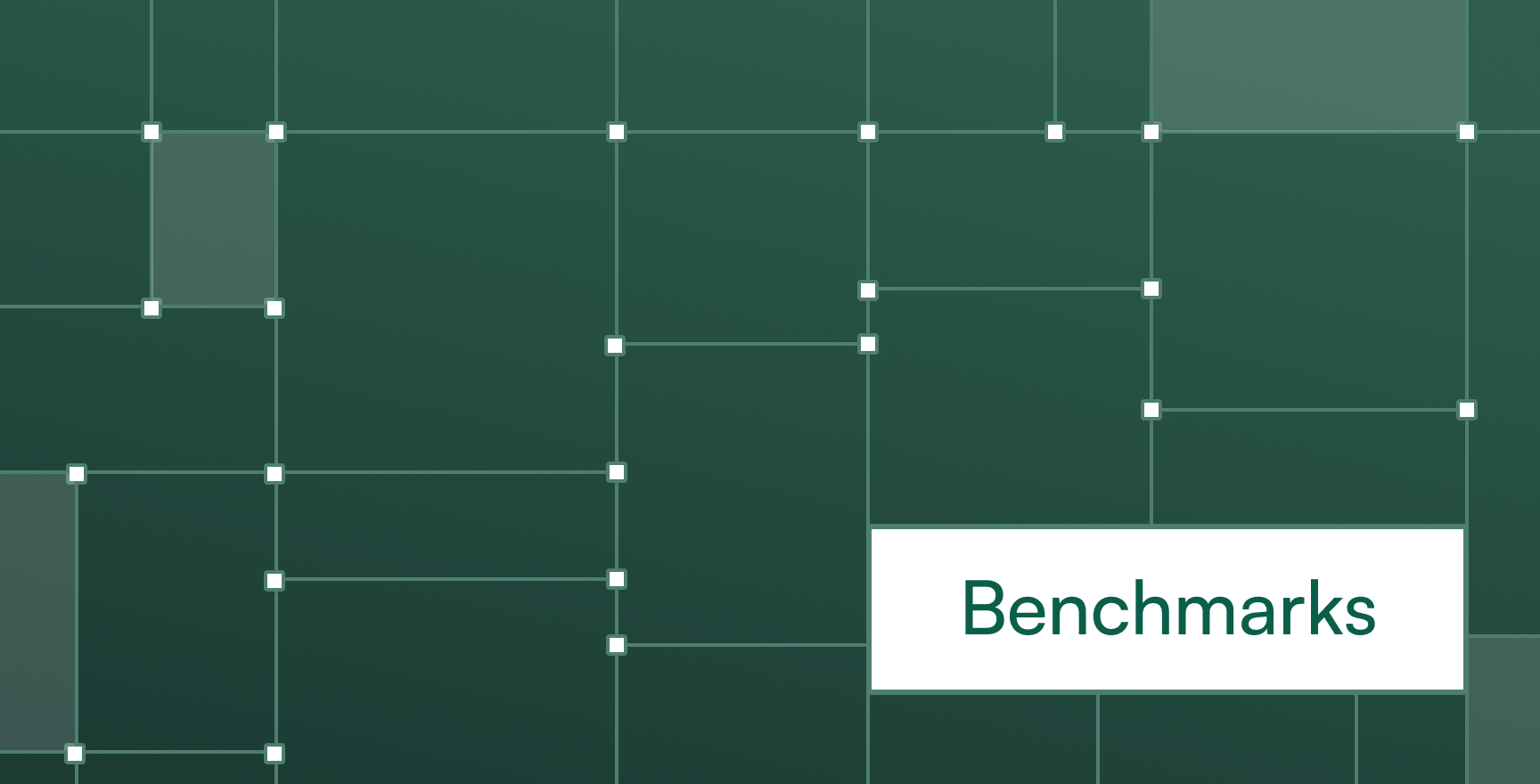
Making Sure AI-Generated Code Actually Works
As tools like GitHub Copilot and Amazon CodeWhisperer become part of everyday coding, we need reliable ways to verify that AI-generated code does what it's supposed to. This is especially crucial in fields like finance or healthcare, where a small bug could have serious consequences.
How We Check If the Code Works
Developers use several approaches to verify AI-generated code:
Unit testing checks individual pieces of code in isolation. For example, if an AI generates a function to calculate mortgage payments, we'd test it with different loan amounts and interest rates to make sure it always gives the right result. Tools like pytest and JUnit help automate this process.
Integration testing looks at how the code works with other parts of the system. If the AI generates an API endpoint for a shopping cart, we'd test how it handles real user sessions, database connections, and payment processing. Tools like Postman and Newman help automate these API tests.
Companies like OpenAI use automated grading systems to test AI models at scale. Their HumanEval dataset runs thousands of coding challenges through models like GPT-4, checking if the generated solutions work correctly.
Real-World Example: Testing a Simple Function
Let's look at how we'd test an AI-generated factorial function:
def factorial(n):
if n == 0 or n == 1:
return 1
else:
return n * factorial(n - 1)
Companies Making This Easier
Several companies are building tools to help verify AI-generated code:
SourceAI focuses specifically on testing AI-generated code across different languages. Their platform automatically generates test cases and checks if the code behaves correctly in various scenarios.
Tabnine and Kite, while known for code completion, also help catch potential bugs in real-time. Their tools analyze code as you write it, flagging possible issues before they become problems.
Looking Ahead
Testing AI-generated code is becoming more sophisticated. Modern development workflows often combine AI coding assistants with automated testing tools. For example, GitHub Actions can automatically run tests on AI-generated pull requests, while tools like SonarQube analyze code quality and potential bugs.
The key is remembering that while AI can write code quickly, we still need robust testing to make sure that code is reliable. As these tools improve, they're making it easier to trust and use AI-generated code in real projects.
Scale your AI Infrastructure
solution faster.
Stop building infrastructure. Start building your AI engineering product.